In recent years, deep neural networks have improved the benchmarks of learning in areas such as vision and speech recognition. This improvement comes with a big price tag. Deep neural networks are very large supervised models and need huge quantities of labelled data at the time of training. In medical image analysis, labeling data is expensive. In certain imaging modalities, such as MRI and CT, 3D analysis and segmentation are required which increases the size of networks and limit our ability to use transfer learning from 2D models of the mainstream computer vision community. I and my team have worked towards reducing the burden of data labeling and improving 3D networks that are specific to medical imaging. On labeling efforts, I will describe our work on producing global and local labels through learning from unstructured text data often available with images. I will then describe a semi-supervised learning approach with a generative adversarial architecture that reduces the need for labelled data in classification. I will also describe our work on a computational framework for network optimization in the context of 3D image segmentation that results in significantly smaller architectures with equal performance to the classical architectures such as UNet and VNet. All these contributions are part of our efforts in the context of developing an AI powered advisor for radiologists.
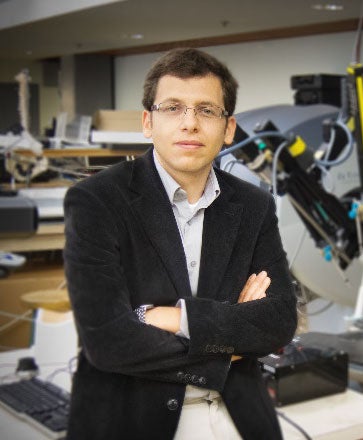
Mehdi Moradi is a research staff member and manager at IBM Almaden Research Center. Over the past five years, he has led a deep learning/machine learning team focusing on medical image analysis. He graduated from the School of Computing at Queen's University, Kingston, Ontario, Canada, in 2008. After completing research fellowships at the University of British Columbia (2008-2011) and at Harvard Medical School (2011-2012), he joined the Department of Electrical and Computer Engineering at the University of British Columbia as an assistant professor in 2012 and IBM Research in 2014. In his research, he addresses the difficulties of deep learning in data limited scenarios of medical imaging were datasets are small and annotations are expensive. He has served as a program committee member of MICCAI and SPIE Medical Imaging and associate editor of several journals in the area, and extensively published in medical image analysis literature.