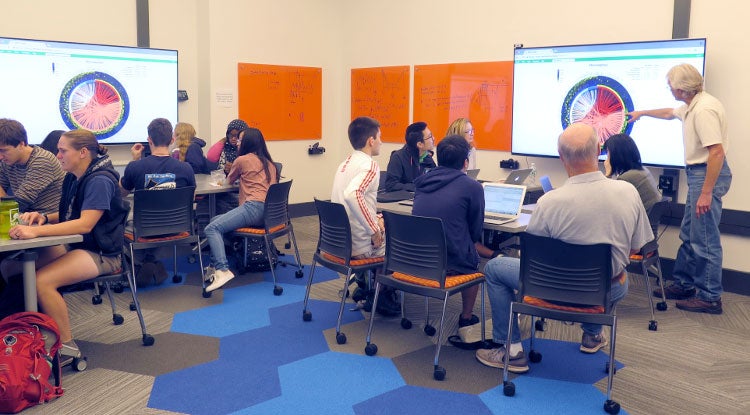
The Rensselaer Health Informatics Challenges in Technology Education (INCITE) Pipeline recruits and prepares students at Rensselaer and worldwide to be data scientists in healthcare using early data analytics courses and experiential research projects centered on real-world health challenges.
With the advent of electronic healthcare records (EHR) and precision medicine, healthcare increasingly relies on health informatics (HI), the philosophy and tools of data science (DS) and their application in healthcare. Rensselaer Health INCITE is a innovative, replicable program that directly expands the health informatics workforce pipeline at the early undergraduate level for students at RPI and worldwide. Health INCITE addresses key challenges in attracting and training top talent:
- The shortage of data scientists
- The lack of awareness among students of HI careers
- The difficulty incorporating reality-driven healthcare projects into curricula due to EHR privacy concerns.
Rensselaer Polytechnic Institute's Data INCITE pipeline for undergraduate data science education consists of an early data analytics course followed by applied data science research experiences on real-world problems. Data INCITE results in data science skills and prompts students to pursue further coursework and careers in data science. Health INCITE builds on Data INCITE, providing a similar pipeline to recruit and train data scientists for health informatics careers.
The Rensselaer Health INCITE Pipeline:
- Produces students skilled in health informatics.
- Creates novel, low-barrier pathways into health informatics for students from a wide array of majors, including pre-med, biology, biomedical engineering, computer science, and mathematics.
- Enables health informatics education at many institutions by creating shared health informatics instructional project resources, including publicly-available, web-based, open source data analytics applications.
- Recruit students to pursue health informatics careers.
The Rensselaer Health INCITE Pipeline has been generously funded by the United Health Foundation
Check out the Project Gallery to see research products (interactive apps, papers, and presentations) created by Health INCITE students in the Data INCITE Lab.
- Health INCITE press release (2018): Rensselaer Polytechnic Institute, United Health Foundation Launch $1.1 Million Partnership to Train Next Generation of Health Data Experts