Attempting to use real medical data in a classroom setting is hard to do without limiting yourself to specific datasets. Through the research being presented we work to create an end-to-end workflow for generating synthetic health data and testing the synthetic data for privacy, resemblance, and utility. This includes creating a novel generation method called HealthGAN and defining metrics for measuring the privacy and resemblance of the generated data. The utility of the data is then measured in the context of the analysis task the dataset was designed to accomplish. The workflow is then tested by attempting to create synthetic versions of two real medical datasets from a secure environment.
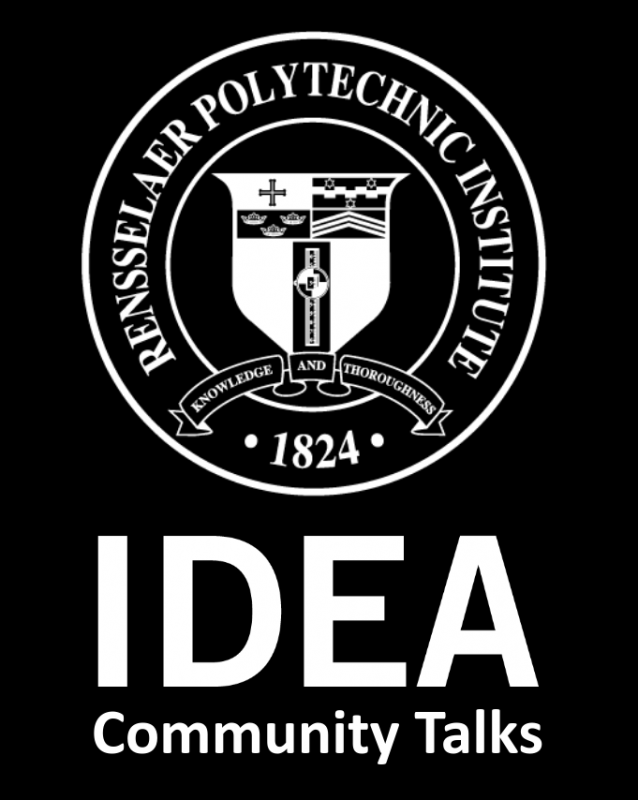
Andrew Yale recently received his Ph.D in Computer Science from Rensselaer. His research focuses on machine learning methods to generate privacy preserving synthetic data. He is advised by Professor Kristin Bennett and works with The Rensselaer IDEA.