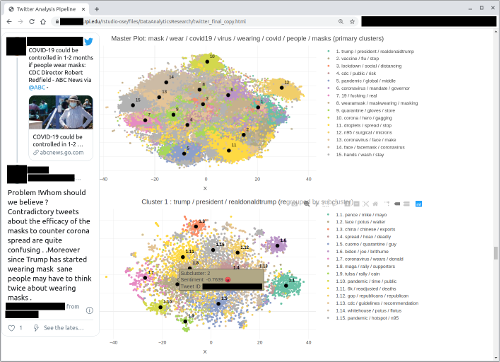
In this exploratory study, we scrutinize a database of over one million tweets collected from March to July 2020 to illustrate public attitudes towards mask usage during the COVID-19 pandemic. We employ natural language processing, clustering and sentiment analysis techniques to organize tweets relating to mask-wearing into high-level themes, then relay narratives for each theme using automatic text summarization. In recent months, a body of literature has highlighted the robustness of trends in online activity as proxies for the sociological impact of COVID-19.
We find that topic clustering based on mask-related Twitter data offers revealing insights into societal perceptions of COVID-19 and techniques for its prevention. We observe that the volume and polarity of mask-related tweets has greatly increased. Importantly, the analysis pipeline presented may be leveraged by the health community for qualitative assessment of public response to health intervention techniques in real time.
- COVID Twitter NLP github: https://therensselaeridea.github.io/COVID-masks-nlp/
- COVID Twitter NLP publications:
Sanders, A. C., White, R. C., Severson, L. S., Ma, R., McQueen, R., Paulo, H. C. A., ... & Bennett, K. P. (2021). Unmasking the conversation on masks: Natural language processing for topical sentiment analysis of COVID-19 Twitter discourse. medRxiv, 2020-08. https://www.medrxiv.org/content/10.1101/2020.08.28.20183863v1 - COVID Twitter Overview Video: https://mediasite.mms.rpi.edu/Mediasite5/Play/28acfc6b54294065ac1a1456f9fadb781d
- Data Analytics Research (MATP-4910 Fall 2020) Final Presentation Video
- Abraham Sanders
- Rachael White
- Lauren Severson
- Rufeng Ma
- Richard McQueen
- Haniel C. Alcantara Paulo
- Yucheng Zhang
- John S. Erickson
- Kristin P. Bennett