Pairwise learning refers to a learning task which involves a loss function depending on pairs of examples, among which most notable ones include bipartite ranking, metric learning, minimum entropy error and AUC maximization. Online learning algorithms consider one instance each time to update the model sequentially, which make them amenable for streaming data analysis. However, most of such algorithms focused on the pointwise learning problems such as classification and regression. A specific challenge in developing and analysing online pairwise learning algorithms is that their objective function is usually defined over pairs of instances which is quadratic in the number of instances. In this talk, we study Learning Theory for online learning algorithm for pairwise learning in an unconstrained setting of a reproducing kernel Hilbert space (RKHS). We present convergence analysis for these algorithms in both regularized and un-regularized settings. The above general online algorithms require to store previous examples which is not memory efficient. We show that, for AUC maximization and bipartite ranking, one can develop a truly online learning algorithm for which the space and per-iteration complexities only depend linearly on one datum. The key idea behind this is a novel formulation of AUC maximization as a stochastic saddle point problem.
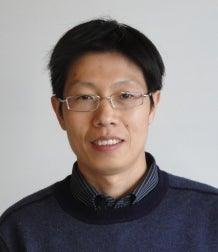
Yiming Ying is currently an Associate Professor in the Department of Mathematics and Statistics at the State University of New York (SUNY) at Albany, USA. He received his PhD degree in Mathematics in 2002 from Zhejiang University, China. He was a a Lecturer (Assistant Professor) in the Department of Computer Science at the University of Exeter (UK) during 2010-2014. Before that, he worked as a Research Associate/Fellow at the City University of Hong Kong, University College London and University of Bristol. His current research interests include learning theory, machine learning and large-scale optimization.